Backfill GA4 Data in BigQuery
GA4 BigQuery backfill ensures comprehensive historical data analysis. This guide walks you through backfilling GA4 data into BigQuery for data continuity and in-depth historical analysis.
Raw GA4 export to BigQuery is not retroactive, and backfilling historical GA4 data is often a necessary step in building a digital analytics data warehouse and reporting system.
Using API for backfilling the data requires technical skills. However, there are other ways to export Google Analytics data, which are outlined in this article.
This article is a tutorial on exporting the data using GA4 data API and loading it into BigQuery.
GA4 data API exports summarized tables, so you must know your desired metrics and dimensions beforehand. Unlike the raw data export to BigQuery, the API export requires understanding the requirements of your eventual staging tables.
1. Enable GA4 data API and set service account permissions
Before starting with data backfill, it is essential to prepare your Google Cloud project, enable the GA4 Data API, and create a Service Account with the necessary permissions.
If you have never worked with Google Cloud Platform, you may benefit from Google’s Quickstart process that creates a new GCP project, service account (named quickstart@), and downloads the API keys in one click of a button. You will still need to give your service account permissions to access GA4 and BigQuery shown below.
Project, API, and Service Account
Google Cloud Project: Ensure you have a Google Cloud project set up. If not, you can create one from the Google Cloud Console.
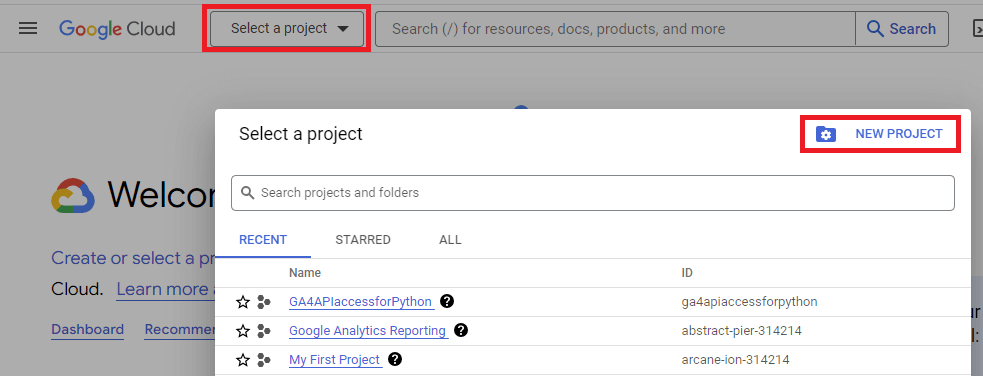
- Enable GA4 Data API: Navigate to the APIs & Services section in your Google Cloud Console. Enable the GA4 Data API by searching for “Google Analytics Data API” and clicking the enable button.
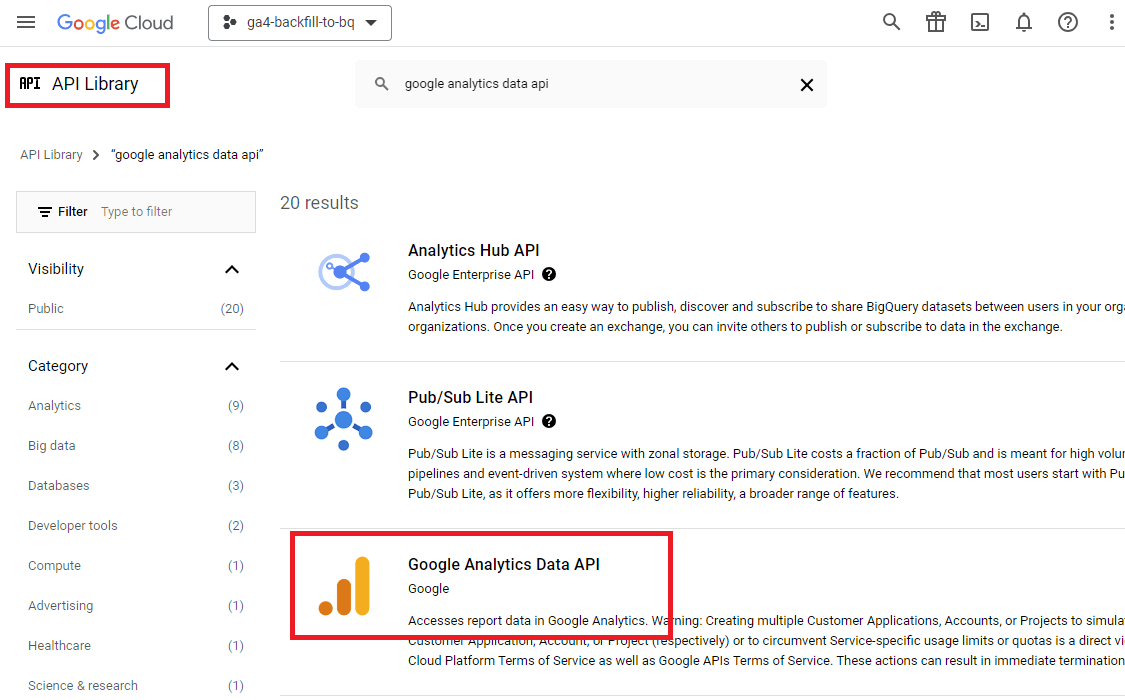
- Create Service Account and API key: Create a Service Account within your project to manage the permissions for accessing GA4 data and BigQuery.
- In the APIs & Services section in the Google Cloud Console click on Credentials and then on Create Credentials -> Service Account.
- Provide a name and description for the Service Account.
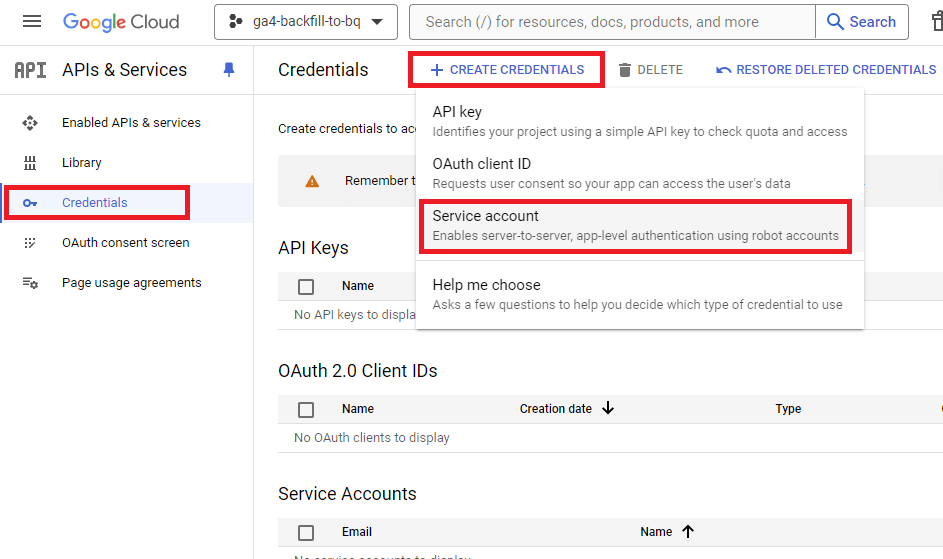
-
- Once the service account is created, click on its name and choose KEYS in the top row. Click on Add Key s at the bottom, and choose Create New Key.
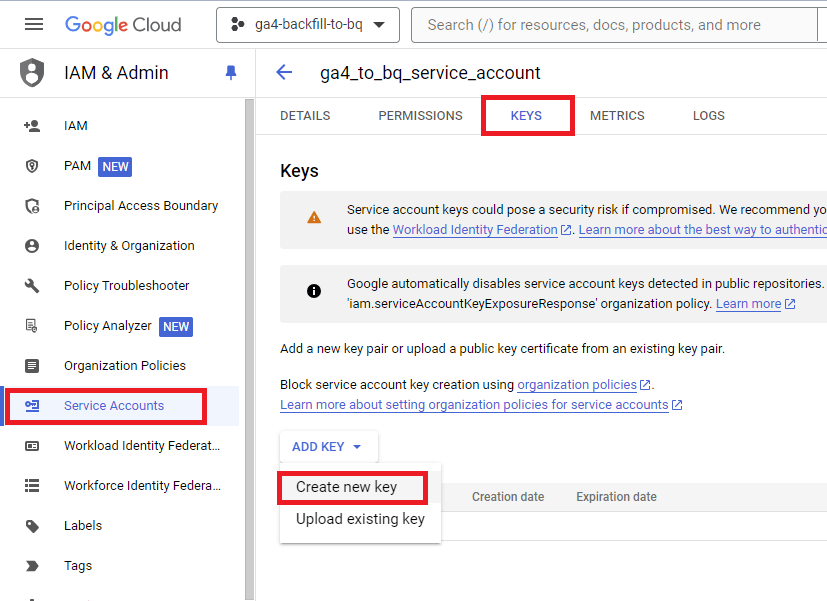
-
- Choose JSON key format, and it will be downloaded automatically.
- While on the Service Account screen, copy its email address. You will need it for the next step.
Permissions for Service Account
Google Analytics
- Open the GA4 property that you are backfilling and navigate to Admin (bottom left).
- Under the Property Setting, click on Property and then on Property Access Management.
- Add your Service Account email and assign “Viewer” role to ensure data read permissions.
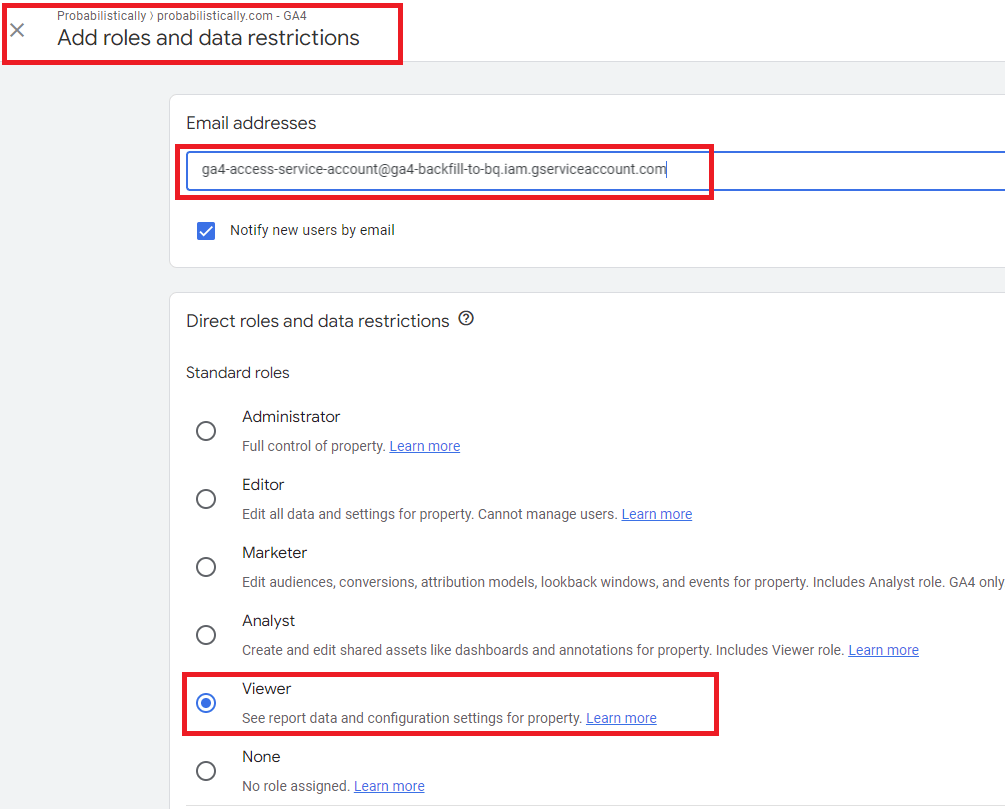
BigQuery access for your service account
- In your Google Cloud project, go to the IAM & Admin -> IAM section.
- Choose Grant Permissions options, and add your service account as a principal with the following roles:
- BigQuery Data Editor
- BigQuery Job User
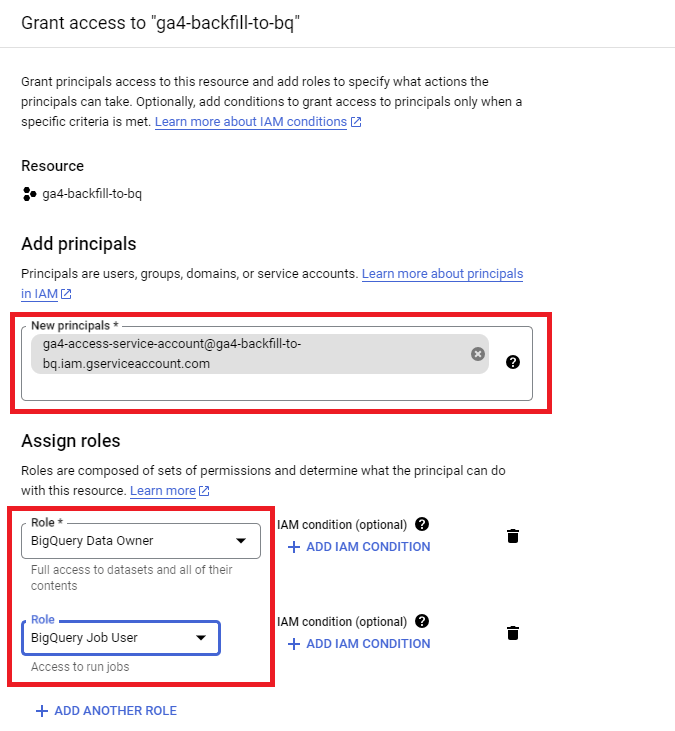
With the project and permissions set up, you’re ready to proceed with exporting GA4 data using the API.
2. Export GA4 data using API
Authorize Service Account in Python/Colab and Install Packages
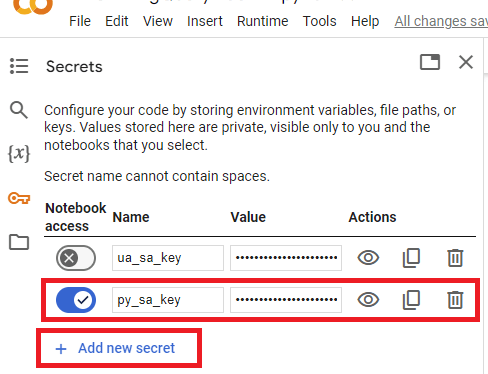
Import these libraries to interact with Google Analytics Data API and process data for your BigQuery backfill.
These variables are crucial for GA4 BigQuery backfill. They identify your GA4 property, manage API call limits, and store service account credentials.
Construct API request, format output, and set pagination
This request structure specifies the timeframe and variables in your GA4 BigQuery backfill output. Adjust these fields to fit your needs using full list of metrics and dimensions available in GA4 Data API.
These functions manage large datasets during GA4 BigQuery backfill, handling pagination and formatting the data into neat dataframes ready for table loading.
3. Load data into BigQuery
Once data is exported and formatted, the next step is to load it into BigQuery. This is a helpful Google guide on the Python BigQuery client for your reference.
If you have not created your backfill dataset (BigQuery term for data schema), you can build it using this function. More on creating BigQuery datasets using python.
Set up your BigQuery environment for GA4 backfill. This code connects to BigQuery and defines where your backfilled data will be stored. For more information, refer to Google’s developer documentation.
# Configure the query job.
job_config = bigquery.LoadJobConfig(
# Set the destination table to where you want to store query results.
job = client.load_table_from_dataframe(
Let’s switch back to GCP, click on BigQuery in the navigation menu and check our data:
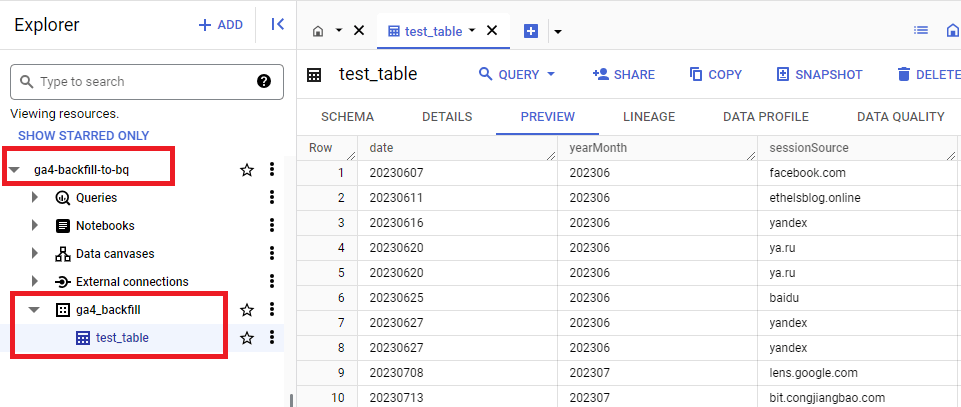
You’ve now backfilled GA4 data into BigQuery, providing a foundation for comprehensive analysis.
The next step is to create the same snapshot of the GA4 data from the raw BigQuery export, so you can use them for unified reporting.
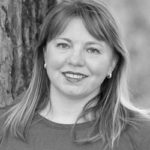
Tanya Zyabkina has over 15 years of experience leading analytics functions for multiple Fortune 500 companies in the retail, telecom, and higher education. She works as the Director of Marketing Performance Analytics for the The Ohio State University. Go Bucks!